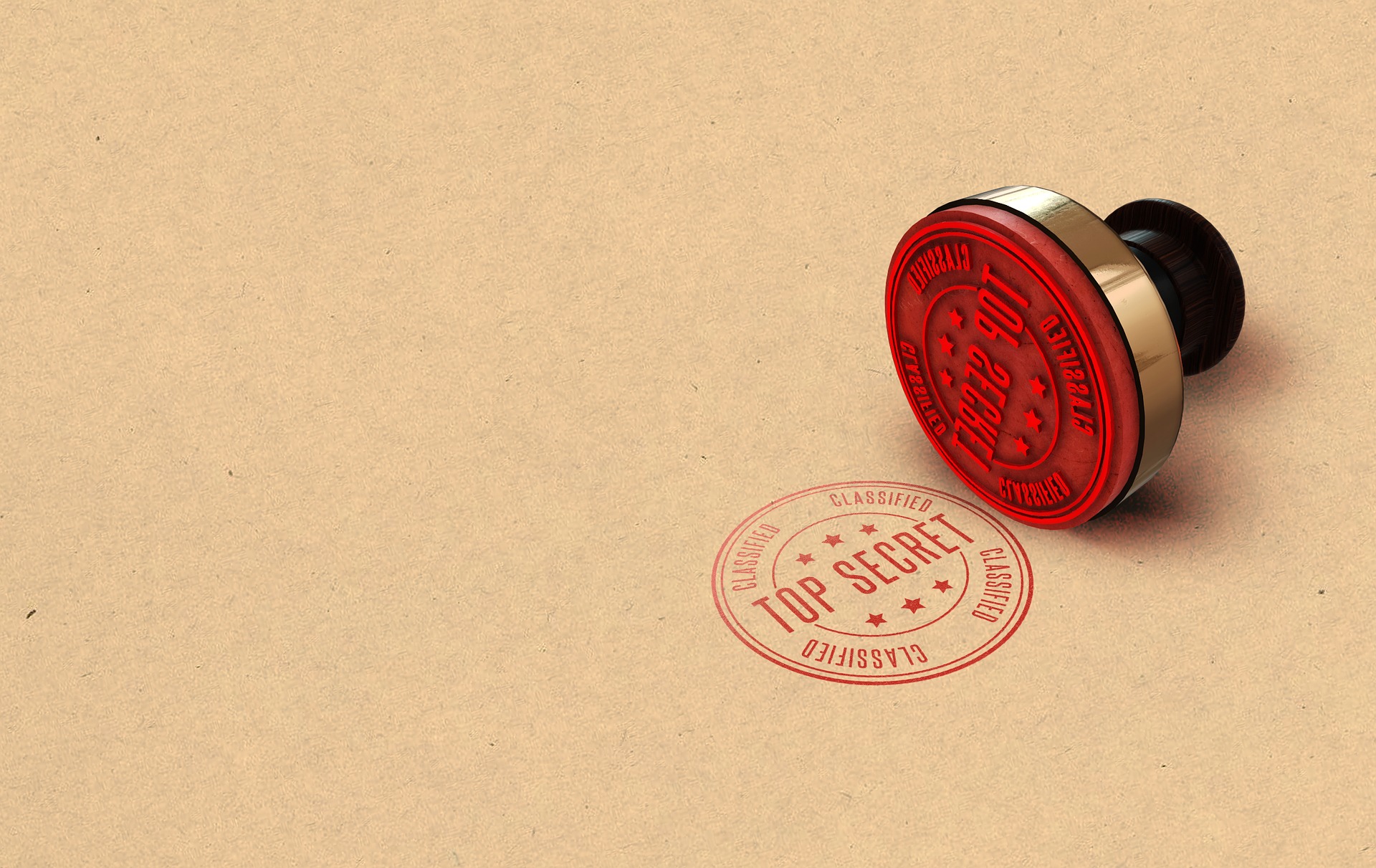
In 2018, the European Union set a milestone for data protection when it enforced the General Data Protection Regulation (GDPR). This legislation made it compulsory for all types of organizations to delete personal data involving European citizens. Compliance with GDPR is now a requirement for all companies dealing with such data, whether they are based in Europe or in other parts of the world.
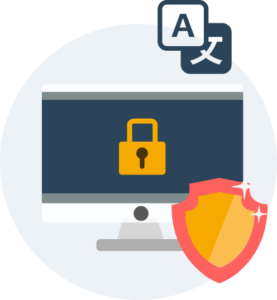
Other countries across the globe have followed suit with their own versions of data privacy legislations. This massive global adoption of GDPR-like laws greatly impacts the language industry. Interpreters, translators, and LSPs worldwide must comply with these regulations whenever they process personal data. In machine translation, data anonymization processes enable the removal of direct identifiers, secondary information, and electronic trails that may disclose personal data and lead to data misuse.
Why Anonymization Matters in Machine Translation
Translation can infringe some of the data protection principles in GDPR. It can violate confidentiality, storage limitation, purpose limitation, and data minimization. To prevent these from happening, anonymization has become an essential part of the translation workflow.
Most documents used by organizations usually contain personal contact data. Aside from this, files shared through digital means can also leave electronic trails. Personal information and even confidential data can possibly be traced from data logged when the files are sent. Then, there’s also the matter of handling official documents with confidentiality clauses. Translating such materials can lead to violations of non-disclosure agreements and confidentiality breaches.
Anonymizing source texts addresses most of the issues in data protection. However, a more complex process of anonymization is required in machine translation. Neural machine translation (NMT) trains on texts with personal data. Translation memory often stores these data without means of deleting them.
By integrating data anonymization processes within the translation workflow, service providers can ensure that machines process data in a secure way that increases translation quality while maintaining compliance with data privacy laws.
How Does Anonymization Work in Machine Translation
Service providers have different ways to anonymize data but the basic steps are essentially similar.
To meet privacy requirements when handling documents, anonymization is carried out before the translation itself. Content from the source is sent to a secure server. It goes through named entities recognition (NER) where key information are identified and classified according to predefined categories.
Once identified, protected data undergo pseudonymization and anonymization. They are replaced by a string of the same type to keep them readable by linguists and machines without revealing personally identifiable information. Other anonymization techniques include scrambling, shuffling, perturbation, and synthetic data generation.
After named entities or protected data are obfuscated, the appropriate language and specialized machine are chosen. Only anonymized data are sent to NMT engines and stored on the platform. The content is processed for translation, then protected data are de-anonymized or replaced with the original data in a secure server. When necessary, de-anonymized data are also localized.
For GDPR compliance, NMTs are not trained with personal or confidential data. Moreover, translation memory stores only anonymized data and not the original ones. Some providers have added security measures in place such as destruction of project files on their secure server after final versions are sent to the requesting parties.
Who Needs Anonymization
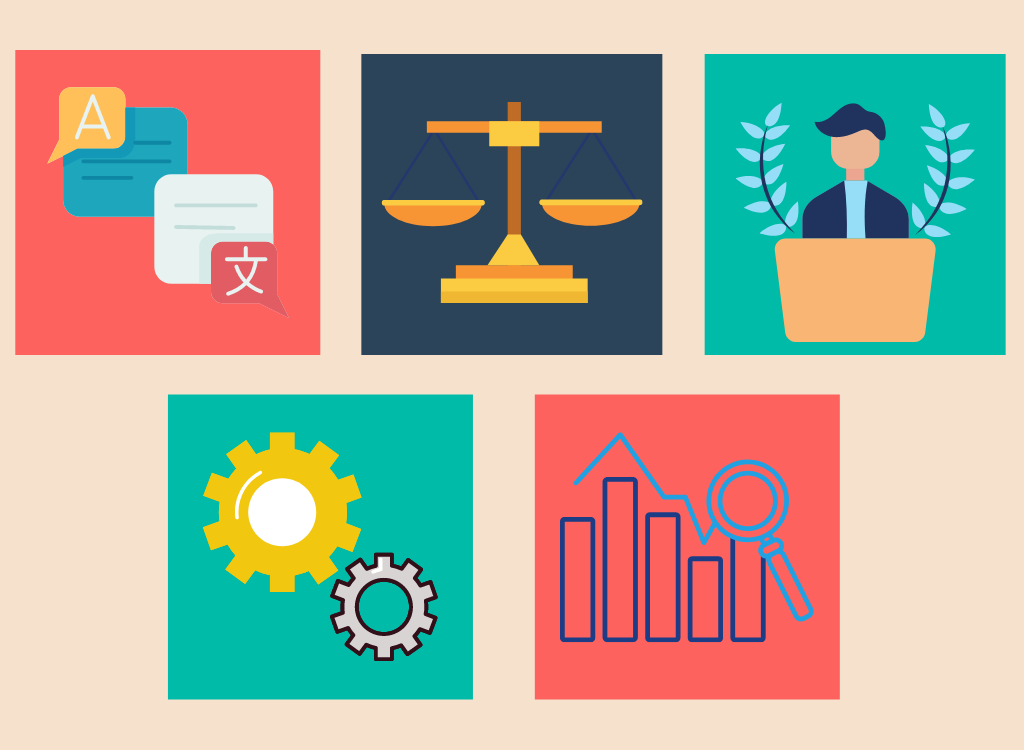
Translation Companies
Aside from compliance to GDPR and other data protection regulations, translation companies must always ensure the safe and secure use of data they process. Incorporating anonymization in their translation workflows enables them to avoid confidentiality breaches and privacy issues. Anonymization also becomes useful when building domain engines across different clients from the same industry, such as three to four clinical trial companies.
Legal Companies building NLP
Companies in the legal sector rely highly on precise language. By utilizing NLP and other AI tools, they can improve the quality of legal work and increase productivity. They use NLP to streamline their research process, draft and analyze legal documents, and process documents in different languages. To keep the language as precise as possible but still protect sensitive client names, anonymization becomes an essential element in any NLP process.
Government bodies
Governments are the biggest manufacturer of data. They build data pools and share these information amongst other government agencies. To keep private and confidential information secure, they must utilize anonymization tools in every aspect of data collection, processing, and sharing.
Highly-regulated industries
Healthcare, banking, finance, insurance, and telecommunication are among the highly-regulated industries that are mandated to keep consumer data protected. Anonymization helps maintain the confidentiality of data and preserve its integrity.
Businesses entities
Businesses, regardless of size, need to share information in the most secure way and to comply with privacy laws. Some require streamlined translation of documents, Excel files, PPT files, terms and conditions, product catalogs, emails, and conversations. When it comes to handling corporate data, we are diligent in ensuring that data on Finances, Taxes, Employees, Customers, Suppliers for Businesses, is protected from outside intrusion or interference while retaining confidentiality and security.
Challenges Faced in The MT Industry
Modern datasets are more complex and high-dimensional. Aside from the massive amounts of data, they are also becoming increasingly difficult to anonymize using standard anonymization methods of generalization and randomization. To fully protect the privacy of entities, service providers must constantly and consistently train machines with high-quality datasets using advanced algorithms.
Another challenge service providers face is the trade-off between translatability and data privacy. The more text is anonymized, the more difficult it is to understand for human translators and machines. This can potentially cause errors and affect the quality of translation. However, if data anonymization is highly effective, it can improve leverage and bring value through the creation of linguist assets that can be reused.
Comments are closed.