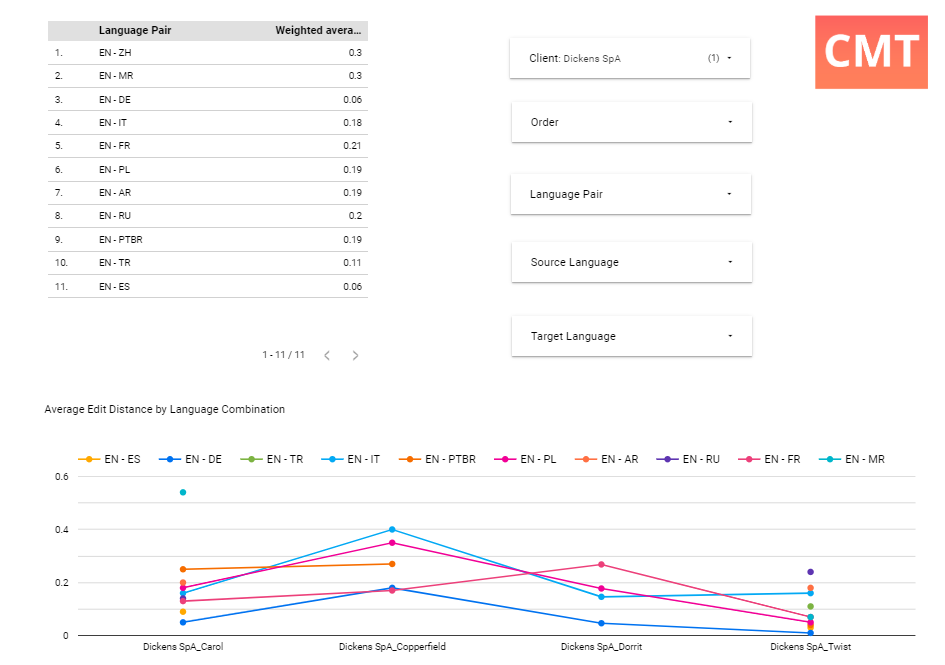
Localization teams in 2024 had a renewed interest in implementing AI translation in workflows. It was a reasonable way to trim their budgets and improve per-word costs. However, just like today, monetization often became a struggle because the mechanism to track human post-editing effort – unlike that of an AI – fairly and responsively is underdeveloped. One way to tackle this challenge is by creating performance dashboards that will show you translation edit distance.
Value for Localization Programs
Machine translation has become more accurate over the years and model accuracy improves with fine-tuning efforts, boosting human review speed. The dashboard is a tool for the localization manager to convert these efficiencies into savings. We developed dashboards that can be used to easily visualize performance by slicing data by language, reviewer, content type, and other useful categories, and by cross-referencing with other metrics, such as editing times, error types, and quality assurance data.
Example 1: Performance by Language
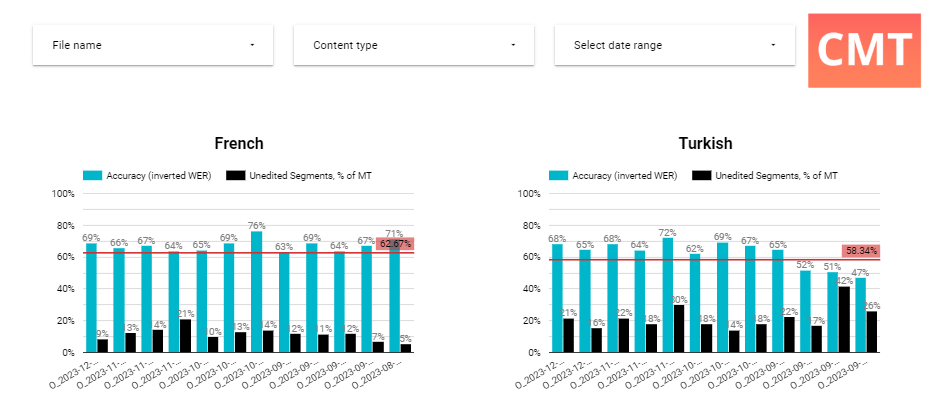
In this view, the localization manager tracks machine translation accuracy in the 3rd and 4th quarters of 2023. For French, the accuracy fluctuates from 71% to 69% unedited words without definitive improvements. The benchmarking red line also reminds the project manager of the expected level of accuracy, giving a clear indication of the MTPE workflow efficiency for each job. For Turkish accuracy begins at a lowly 47% and improves to 68% in December. This informs the project manager’s action plan: to adjust the costs for Turkish and start working on French model accuracy.
Example 2: Performance by Translator
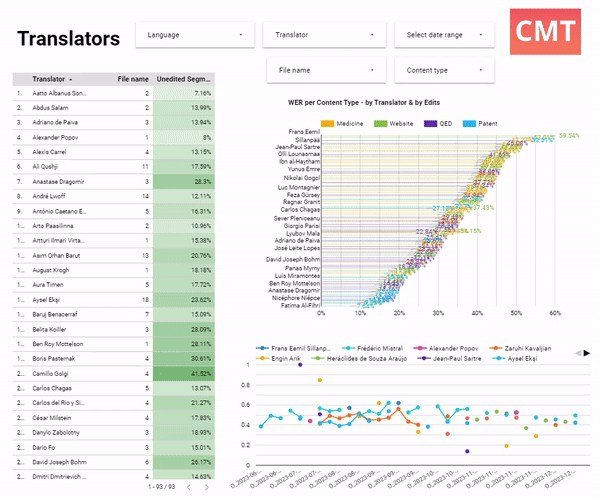
Here, a project manager is reviewing AI performance by linguist. They all work in the same domain of medical translation and use the same AI model. Two linguists clearly sit on opposite ends of the productivity range: Francoise edits very thoroughly and changes more than 38.32% of words, while Alexia is very fast, and only corrects 20.34% of the original text. The bottom chart shows that from June to December, the edit distance has been on the rise for almost all translators. This is a call to action for the project manager: they may want to look at the MT model performance, and spot-check Alexia’s work, since she was editing much less than anyone else on the team.
Two Reasons Why Localization Teams Struggle with Savings
Since 2018, the growth of machine translation review has surged without establishing a standard monetization approach, leading organizations to adopt varied strategies. Strong negotiators, like top-10 language service providers (LSPs), can leverage their position for substantial cost reductions, whereas smaller buyers often see minimal benefits. Disparities in translation management systems, such as differing methods in Trados, memoQ, and Smartling, complicate consistent monetization of AI benefits due to incompatible tracking metrics. While some organizations like TransPerfect, Welocalize, Dell, and Acolad have developed proprietary tools to gauge review efforts, most of the market still grapples with these issues.
Machine translation review has grown immensely since the first neural models in 2018, but no industry standard on how to monetize it has emerged. Debates rage on, and every organization follows a different approach. It’s the law of the jungle.
Translation buyers looking to convert technology efficiencies into tangible savings face tough negotiations. Strong negotiators like top-10 LSPs can easily replace suppliers, and thanks to a stronger negotiation position they achieve a 40-65% reduction in costs. Vulnerable buyers, in particular, one-person localization teams serviced by a single vendor in control of linguists, connectors and TMS may get nothing at all.
The second challenge is technical. Popular translation management systems (TMS) have mature functionalities to save resources with translation memories, but monetization tools for machine translation monetization are too basic. They work on a per-project basis and use different tracking units across various products. For example:
- Trados plugin Post-Edit Compare uses 10% bands
- memoQ post-translation analysis differs from Trados with a Translation X category
- Phrase Post-editing analysis uses different default bands from Trados and memoQ
- Smartling provides translation error rate (TER) scores instead
Post-editing analysis can only be run within the specific system and can’t be compared with data coming from other software without some conversions. Discrepancies delay the day when a widely accepted method of monetizing AI benefits may be established.
Individual savvy organizations like TransPerfect, Welocalize, Dell, and Acolad have developed proprietary tools to measure the review effort and its compensation. For the majority of the market, it’s still a problem.
How to Build Your Dashboard: Getting Raw Data
There are two ways to get performance metric data: either pull a ready-to-use analysis from the translation management system or calculate independently from the TMS by comparing files <before> and <after> review.
Most of our clients have elected to pull calculations from their TMS, because they use only one TMS, and it already takes care of computation. Clients using memoQ pull AI translation edit distance reports, while Smartling users export String Changes Report as a CSV, and our script can then convert both into a database with the desired structure.
Independent comparisons based on Xliffs is practical for organizations working with several different TMS tools and looking to standardize.
For visualization of the data, we use Google Looker Studio, a free tool to craft dynamic dashboards that automatically synchronize with the database created earlier. In a Microsoft-powered organization, it is possible to use Microsoft Power BI or Tableau.
Selecting Metrics
The scientific and quirky world of machine translation teems with 900+ metrics, the most popular of which are BLEU, WER, TER, and COMET. There is no perfect metric, and each of them requires detailed scientific explanations (“n-grams”, “pre-trained language models”), and all of them collectively face regular criticism. The world of linguists is far removed from these debates, it’s not realistic to negotiate rates based on BLEU and COMET points.
Here are the metrics that we have found useful in business:
- Accuracy in % – a simplified percentage is easy to understand and communicate. To get a % of accuracy, we track words that remain unchanged from the machine translation output to its post-edited version.
- Edit distance in % – it’s possible to track it in two units: either edited words (WER – word error rate) or edited characters (TER – translation error rate). Tracking words has the advantage of being fairly uniform across different languages regarding the average length of the word. One character means more in Japanese than it does in German. NB: we prefer to invert the edit distance and call it “Accuracy” because a straight percentage score promotes understanding and acceptance across groups of people.
- Unedited Segments – sentences that were not changed by linguists. They may be billed in the same way as 100%-matches in translation memory.
We slice the data into four views:
- Performance by reviewer. Language is subjective, and some translators overedit, while others skim through the content. Productivity comes from the combination of human skill and engine accuracy. Using a view by translator, a localization project manager may train translators, and detect fraud.
- Performance by domain/content type. Tracking the edit distance difference across different content types allows for monitoring quality levels and detailed profitability of MT for each topic. Creative and marketing texts, for example, require more editing effort to achieve the emotional effect on the reader.
- Performance by client. Language services providers find it interesting to track edit distance per client to achieve margin targets.
- Performance over time. This monitors gradual improvements to models and post-editing skills and highlights sudden changes.
Template Performance Discount Table
The translation and localization industry has not produced a standardized discount table yet, so you’re free to propose your own. We offer a template below that proposes mild discounts and splits the benefits between the buyer and the supplier. The supplier needs to be a beneficiary of increased productivity as well as the buyer. Without reviewers agreeing to adapt to improved AI, there can be no efficiency, and the whole supply chain stagnates.
Accuracy Inverted WER | Discount from translation rate | Review speed words per hour | Vendor Income per day |
<50% | 0 | 250 | 100% |
50-59% | 10% | 300 | 108% |
60-69% | 20% | 400 | 128% |
70-79% | 30% | 700 | 154% |
80-89% | 40% | 900 | 180% |
90-99% | 50% | 1200 | 210% |
Afterword
The year 2025 in localization, much like 2024, begins as the year of improving efficiency of AI-automated tasks. Localization teams face stronger pressure than ever to do more with less, or to replace human translation with Generative AI.
We hope that this guide on building metrics and dashboards for AI translation performance will help localization teams find the best combination of model and human to succeed in challenging times and protect their teams from replacement. And for the more advanced and personalized solution you can always reach out to our experts at https://custom.mt/book-a-call/
Comments are closed.