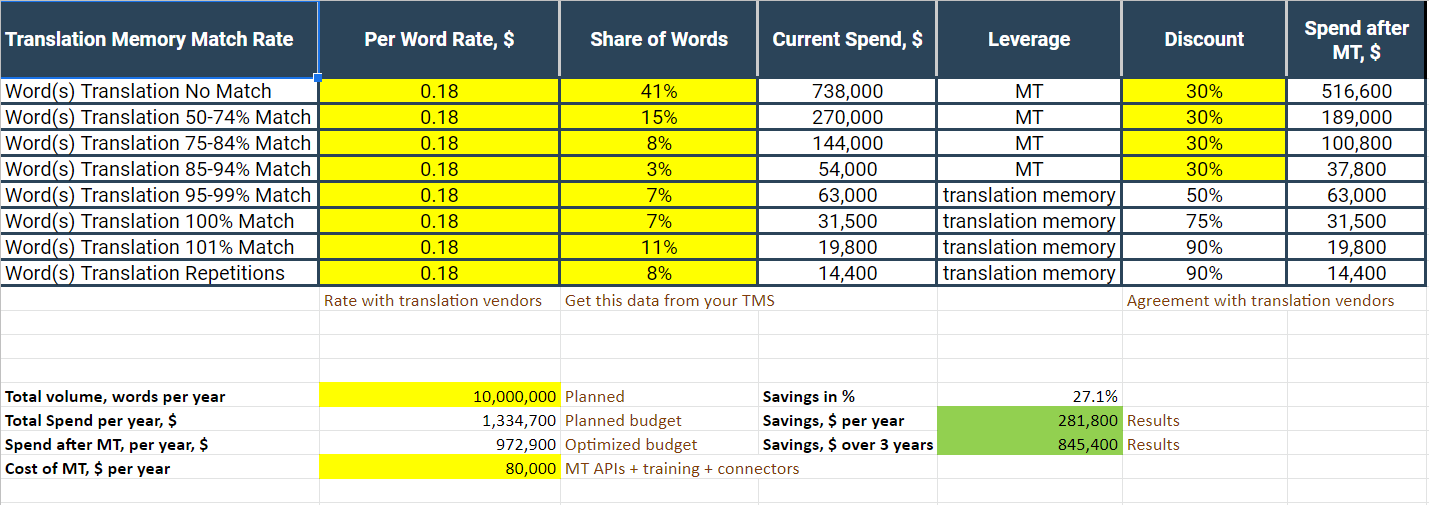
This Excel template is designed to help translation managers calculate savings from moving parts of their translation budget to machine translation post-editing workflow. The table factors in the translation memory, repetitions in the text, and different levels of discount depending on the match rate. With some patience and persistence, you can not only save up on the cost but also adjust for differences in translation rates by language.
Example Calculation – Acme Engineering
Download the template here.
In our example calculation, the fictional company Acme pays $0.18 per word to a translation company. With a volume of 2 million source words per year and 5 target languages, the work costs Acme $1.3 million USD on translations, which they optimize in the course of this project.
They already have translation memory in place, and decided to keep using it for matches above 85%. Next, they invest 3% of their budget into machine translation and pass fuzzy matches through MT with human review. Based on the MT accuracy scores, they negotiate a 30% rate reduction with their supplier for MTed segments. Following the implementation, they start seeing savings, which come up to 27% of the budget. Acme sees an economic benefit of a million dollars over three years.
Word rate.
This is your negotiated per-word rate with your suppliers. It often varies per language because of the different costs of living in various countries. To estimate savings in a machine translation program with many variables, divide the cost options into 3 tiers and calculate the savings individually per Tier. Example tiers:
1. Japanese, Korean, Nordic – $0.20 per word
2. German, French (medium) – $0.15 per word
3. Spanish, Turkish, Portuguese – $0.09 per word
Share of volume.
This is the split of your incoming translation work by discount level for the translation memory fuzzy matching. You can obtain the information on the split from your translation management system (TMS) by adding past analyzes or by tasking your translation company with compiling these numbers. If you don’t have any discounts in place yet, this will be a great starting point to negotiate them.
Current spend.
This column represents your translation budget sliced by the translation memory match rate. It will be populated automatically based on a formula including your discounts and words sourced.
Leverage.
Indicates which technology pre-translates incoming sentences, translation memory, or MT. As we stated earlier, a typical cut-off for MT is 85%, but if your MT is very accurate, go bolder, and target 95%.
Discount.
MT discounts are a hot topic for debate; they are negotiated at various levels in real contracts. Great places to check discount levels are Proz and Smartcat marketplaces, where freelancers often indicate their pricing for translation and post-editing separately.
Here is our guidance on translation discounts based on MT accuracy:
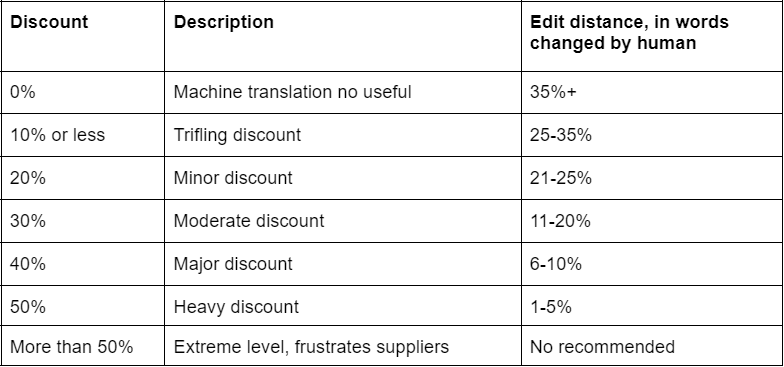
When negotiating MT discounts, it’s always great to have edit distance and machine translation scores on hand to make sure that the discussion is driven by data.
Spend after MT. The projected change in spending after MT discounts are implemented. Segments populated from the translation memory do not change, but the spending for MTed segments will go down.
Important Considerations
Edit distance biases. Machine translation quality varies from project to project, and edit distance is often influenced more by the personality of the reviewer. Some people rewrite minor details in the translated text for stylistic coherence or personal preferences. Others prefer to go fast and ignore minor discrepancies in favor of expediency. Track edit distance across several people and adjust for bias of individuals whose performance is wildly different from others in the calculation that affects rates.
Ramp up MTPE. Machine translation savings do not happen overnight; in most cases, they require vendors to get used to the engine output and learn to recognize typical mistakes made by the machine. While our calculation portrays savings as instantaneous, in your real-life scenario, it might take a couple of months, or even longer for MT benefits to kick in.
Operationalizing MT is about change management. While it is extremely important to have top quality machine translation and best-in-class models, the savings come from changing existing business relationships and convincing suppliers that even though they earn less per word with you, they will in the long run win more per year. Bank on optimistic suppliers who embrace MT ahead of others, and direct more volume their way. The person in charge of MT program should be a diplomat and a trainer; someone savvy in working with people, rather than software.
More impact from MT
Machine translation’s economic impact just begins with the optimization of the translation budget. There are more than 50 other use cases where MT can bring an even greater benefit to your organization, including these.
1. User chat to allow one customer team to support all locations
2. Information monitoring
3. Email
4. Internal communications
5. Video archives
6. Multilingual forums
7. Review translation
After you complete the implementation in localization, move on to the next use case and help foster more multilingual communication inside your organization.
Comments are closed.