On January 28 we called for language industry peers to evaluate the new large language model Deepseek for translation tasks. More than 30 volunteers came forward, and the first results have already come in. We’re hoping to have at least 10-15 language combinations evaluated within the scope of this expercise.
We will publish information as it becomes available, and provide a full report next week.
# | Language Combination | Useful translations | Evaluated by |
---|---|---|---|
1 | English to Turkish (Scientific) | Google – 89.13% GPT-4o – 84.78% DeepSeek – 84.78% |
Sıla Alan, master student at Heidelberg University |
2 | English to Turkish (Legal) | DeepSeek – 96.30% Google – 96.30% GPT-4o – 92.59% |
Sertan Ceylan, CEO at Pilot Translations |
3 | English to Polish (marketing website) | DeepSeek – 87.84% Google – 78.38% GPT-4o – 75.68% |
KONTEKST Language Operations |
4 | German to English | Google – 46.97% GPT – 43.94% DeepSeek – 42.42% |
Giles Tilling Translator and Copywriter, Wordworks |
5 | French to German | DeepSeek – 63.33% GPT-4o – 60.00% Google – 50.00% |
Matthias Caesar, partner at iLocIT! |
6 | English to Indonesian | DeepSeek – 96.00% Google – 94.00% GPT – 94.00% |
Miranti Cahyaningtyas Language professional |
7 | Czech to Hungarian | GPT – 100% DeepSeek – 100% Google – 100% |
Petr Sedlacek Co-founder at LOCO |
8 | English to French | Google – 91.43% DeepSeek – 88.57% OpenAI – 80.00% |
Adil Boussetta Expert linguist, Translation Technology Consultant |
9 | French to Italian | DeepSeek – 96.30% Google – 94.44% OpenAI – 94.44% |
Francesco Saina, Translator, Interpreter, Researcher |
10 | English to Japanese | Google – 44.74% DeepSeek – 31.58% OpenAI – 18.42% |
Kaori Myatt, Linguist and SEO specialist |
11 | English to Spanish | DeepSeek – 89.84% OpenAI – 86.72% Google – 84.38% |
Laurie Hartzel for MathWorks, Senior Lacalization Expert, Language quality expert |
12 | English to French | DeepSeek – 83.59% Google – 80.47% OpenAI – 78.13% |
Myriam Bocquillon for MathWorks, Senior Lacalization Expert, Language quality expert |
13 | Swedish to Norwegian | Google – 97.06% OpenAI – 95.59% DeepSeek – 92.65% |
Jonas Lundström Business developer |
14 | English to Arabic | OpenAI – 96.83% DeepSeek – 92.06% Google – 90.48% |
Najat Keaik Translator, Project manager, QA specialist |
15 | Turkish to Russian | DeepSeek – 91.46% Google – 90.24% OpenAI – 86.59% |
Olga Hergül Localisation program manager |
16 | English to Italian | OpenAI – 88.14% DeepSeek – 86.44% Google – 83.05% |
Elena Murgolo Senior localisation consultant, Language tech specialist |
17 | German to Italian | DeepSeek – 88.89% OpenAI – 83.33% Google – 80.56% |
Elena Murgolo Senior localisation consultant, Language tech specialist |
18 | English to Spanish (Latin America) | Google – 100% DeepSeek – 100% OpenAI – 100% |
delsur. |
Evaluation Method
Volunteers sent us samples of texts they usually work with in professional translations. We segmented the texts based on punctuation and translated sentence-by-sentence with 3 models:
- Google Translate
- Deepseek R1
- OpenAI GPT-4o
Each sentence in the test file has been shown to linguists three times, once per model. Model names have been hidden from the evaluators to avoid bias towards any specific model.
Evaluators then scored each translation from 1 to 5:
-
1 – Catastrophic: the translation is incomprehensible or contains mistakes that could put lives in danger or heavily damage the reputation of the company/author
-
2 – Inadequate: the translation includes errors that seriously affects the understandability, reliability, or usability of the content
-
3 – Passable: the translation is awkward or partially incorrect but overall comprehensible
-
4 – Good: the translation contains some minor errors that do not seriously impede the usability, understandability, or reliability of the content. Also, most of the meaning is reproduced and the language is fluent
-
5 – Perfect: no errors and good fluency
“Useful translations” in the table refer to scores from 3 to 5, combined together for an easy to read single number. We will provide more detailed distribution in the full version of the benchmark.
Model
We opted to use the largest model Deepseek R1 for the test.
– DeepSeek-R1-Distill-Qwen-1.5B
– DeepSeek-R1-Distill-Qwen-7B
– DeepSeek-R1-Distill-Llama-8B
– DeepSeek-R1-Distill-Qwen-14B
– DeepSeek-R1-Distill-Qwen-32B
– DeepSeek-R1-Distill-Llama-70B
Our experience during testing was that the smaller models hallucinated a lot and provided subpar translation quality compared to the flagship.
Prompt
Our testing revealed that DeepSeek R1’s reasoning capabilities require a different prompting strategy compared to traditional translation models. The model’s distinctive feature is its ability to imitate human reasoning within <think></think> tags, which significantly influences the final translation output.
After several iterations and experiments, we developed the following prompt template:
messages = [ { “role”: “user”, “content”: “You are a professional translator. Translate input text into {target_language} while preserving all original formatting, style, and special characters. Important: No explanations or comments in your output – just translation!” }, { “role”: “user”, “content”: “source_segment” } ] |
We deliberately split the instructions and source text into two separate user messages to prevent the model from generating unnecessary disturbing separators (like “## Output:” or “**Translation:**”)
The prompt emphasizes preservation of formatting and special characters while maintaining a clean output without explanatory text.
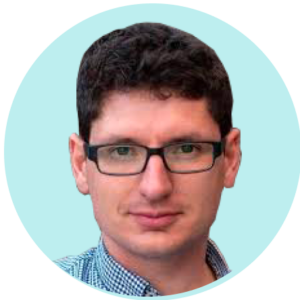
Comments are closed.