In this case study, our client is a medium-sized translation company in Moscow that specializes in the medical field. They are a regional leader in medical documentation, with tons of clinical studies, pharma labels, and Covid-19 announcements. The agency has experimented with a stock Yandex engine and contracted Custom.MT to see how far the improvement of English to Russian machine translation can go with training.
Language combination: English to Russian
Domain: Medical
Training dataset: 250k segments
Highest BLEU score attained: 43 (above average)
Gains over stock engine: +31% segments that need no editing
The project gripped us with challenges from the first week. Over 15 years in business, the client has accumulated 1.1 million parallel segments in the subject matter area. When we looked at the size of the dataset, it made our eyes water with excitement and anticipation: this is one killer TMX to contend with.
Our production team was also wary of the inflectional character of Russian, which means word endings change depending on gender, singular/plural, and cases. Machine translation engine needs to take it into consideration. Otherwise, editing all these suffixes can take as much time as translating from scratch. On the line of difficulty, Russian is a harder nut to crack for MT than French and Italian but thankfully not quite as difficult as Korean and Turkish.
MT Engine Training
First, for the proof of concept project, we splintered a part of the monster TMX with the most relevant and high-quality translations, processing 0.35 million parallel sentences. From our previous Medical project, we knew that combining too many areas of Life Sciences together can be harmful to training. You don’t go to your allergologist for a knee operation. Likewise, you don’t train Covid engines with optometry. After selecting a proper sample, we cleaned it up with our usual data pipeline operations and uploaded it into 4 different MT consoles. Once training had run its course, we measured BLEU scores.
Providers | BLEU |
Trained MT Engine 1 | 43.27 |
Stock MT Engine 1 | 34.34 |
Trained MT Engine 2 | 37.38 |
Glossary MT Engine 2 | 29.67 |
Stock MT Engine 2 | 31.72 |
Stock MT Engine 3 | 35.4 |
MT Engine 4 | 32.80 |
Stock MT Engine 5 | 24.19 |
Already at this stage, it was clear that E4 and E1 perform on a similar level, but training spices things up. E1 improved the score by 26% after we loaded it up with data. Training E2 gave the engine an 18% better performance. These were good initial results to be checked by an actual human evaluation. By contrast, E5 did not show improvement post-training in this project, so we eliminated it from the project’s next stage.
Human Evaluation
E1, E2, E3, and E4 made it into the human evaluation phase in this project. For the evaluation, the translation company provided three specialist medical translators who scored segments according to our methodology. They marked them with either Perfect or Good if the segments were good enough to leave them without edits.
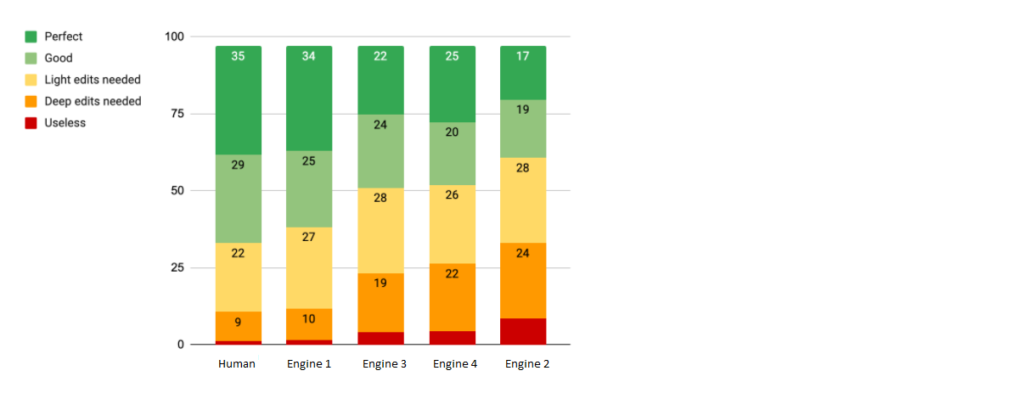
MT did not beat human translation this time but caught up really close with a 4-point difference. E1 gained 59 of these green points, much better than 45 of the stock E3.
Gains:
- 70% faster editing
- +32% in BLEU
- +31% good and perfect segments that need no editing
E2 scored poorly even with training this time, as was rated the last by every reviewer. E4 and E3 vied for second place after E1, and opinions were split.
Engine preference by reviewer
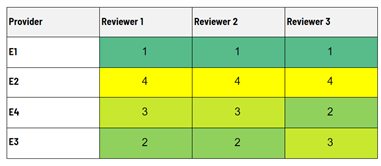
Since the translation company is using Memsource and Smartcat as TMS/CAT tools, we were limited in the choice of technologies to only what is already integrated into Smartcat. Memsource naturally has the largest pool of MT integrations among CAT-tools, while Smartcat is only beginning to add engines with training capabilities.
E1 winning on both BLEU scoring, human evaluation and integrations simplified our final recommendation. We picked E1 and configured it in the client’s workflows. The first live projects are already running with trained engine support.
Case study by Konstantin Dranch
Comments are closed.