Case study
In this case study, we look at a machine translation engine training project with a French LSP. We trained a set of MT engines and evaluated the performance both automatically and with a human eye with the client’s pool of linguists.
Language combination: French to English
Domain: Financial documentation
Training dataset: 607k parallel segments (295k after cleanup)
BLEU scores attained: 44 and 43
There was a “Kasparov vs Deep Blue” moment when the machine won against a specialist human translation 5 out of 5. In our test, five specialist translators ran a blind test on a group of engines, among which human reference was hidden as another MT output. Without knowing which was which, the translators scored 96 segments for each engine from 5 (Perfect) to 1 (Useless). By the number of segments that needed no editing, the linguists placed human translation only in the 3rd position.
The results
The trained engine gained a lot on every metric compared to the very strong stock engine from DeepL that the client used before:
- +30% in the human evaluation score
- needs 42% less time to edit
- need -62.5% effort (WER) to edit
The company is now implementing a new compensation scheme. Meanwhile, we’re analyzing mistakes still made by the machine to retrain it a couple of months down the road.
Error types
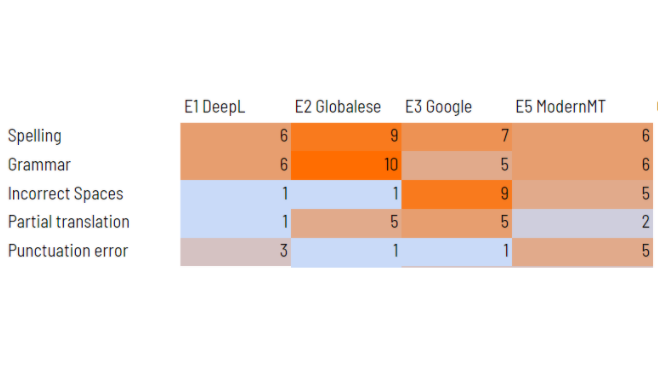
We estimate that the savings from upgrading to a trained engine will improve the LSP’s gross margins by more than 10% in 2021.
The machine or human translation debate does not always end like that. In another evaluation, this time for English to Russian, people won against every engine trained. Russian is a harder nut to crack for MT because it is an inflectional language. However, it still makes sense to train. The difference between stock and the best performing trained engine was huge; the client still gained +60% better MT performance after training.
Comments are closed.