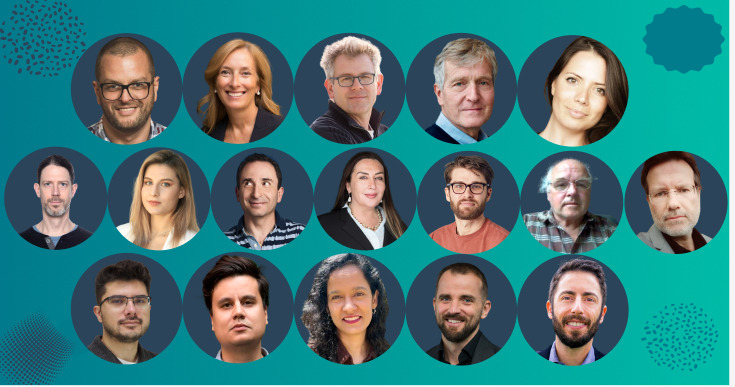
The second event in this series highlighted the localization industry’s quick adoption of ChatGPT, GPT-4 and other large language models (LLMs). Compared to the shock and surprise in February of this year, this time participants saw action across the board.
Over 3,000 watched the event live and in recording later.
The event featured panel discussions on five key topics: news & trends, buy-side pilots, research, integrations in translation management systems, and advocacy advice. Each discussion provided valuable insights into the industry’s current state and highlighted how new technology shapes its future.
We have summarized the main takeaways from each session in the article below.
Buy-side Pilots in Localization Teams
Moderated by Mirko Plitt (WHO Academy), this panel included Indira Lorenzo (Flo Health), Michael Levot (Canva), and Soeren Eberhardt (Microsoft).
Localization Case Study: Flo Health
Indira Lorenzo
Director at Localization
Flo Health
Flo Health Inc. is a women’s health app in the wellness and fitness industry. It offers personalized insights to help women understand their bodies better.
Overview:
- Flo Health has a huge library of information and is working towards making it available in different languages.
- Benchmarked GPT 3.5 for translation customizing the model via prompt engineering.
- Tested linguistic and medical accuracy with human experts.
- Based on medical accuracy evaluation, Flo team is inclined to use machine translation models and not general-purpose GPTs for now. MT offers a lower risk of hallucinations in content that deals with health.
Recommendations
- The goal for GPT Pilots is to bring everyone on board. Indira: I’d also recommend that stakeholders involved conduct thorough testing. This makes our work more meaningful and impactful.
Localization Case Study: Canva
Michael Levot
Localization Program Lead at Canva
Canva is a graphic design platform that allows users to create social media graphics, presentations, posters, documents and other visual content. It offers thousands of templates and designs for users to choose from.
Overview:
- Michael Levot demonstrated a project in language quality assurance. He increased the capacity for LQA at Canva without increasing the budget.
- Canva has LLMs implemented in the product as well.
- Canva trained the Davinci base model with 1,000 correct and incorrect samples and fine-tuned the model. Before LLMs, we had an 8% yield, and after using fine LLMs, we realized a 76% yield.
Localization Case Study: Microsoft
Soeren Eberhardt
Global Site Manager at Microsoft
Key Takeaways:
- Currently, there are many opportunities for conversational bots. These bots are ideal for customer service, especially when the bot is customized to meet the needs of a company’s product and client base.
- The industry at large needs to keep its expectations realistic. This means understanding the limits of ChatGPT and what it can and cannot achieve.
- There are a lot of valid concerns beyond the fabrication, especially regarding data privacy, cost (when using the models), and information quality.
- On recommendations, the focus should be on using ChatGPT as a tool for content creation in different tools instead of a tool that can be inserted into translations. This means we ought to use these tools to make our work better.
Innovation Showcase
This panel section reviewed innovations from tech companies companies with OpenAI integration. Madhu Sundaramurthy from Summa Linguae moderated this session.
ChatGPT integration by Lokalise
Lokalise is a cloud-based translation management system that helps businesses automate localization processes. The presentation showcased an integrated GPT for string rephrasing, shortening, and glossary morphology.
ChatGPT integration by Custom.mt
Custom MT is a machine translation implementation company. The integration brings GPT-4 for translation into:
- Trados
- memoQ
- Smartling
- Shopware
With GPT-4 translation, the linguist can define a glossary and a style guide, and have the translation model adhere to them. For example, Stacey Lisina demonstrated a use case with gender-sensitive translations.
ChatGPT integration by Bureau Works
Bureau Works is a cloud-based translation management system. The presentation showcased how Bureau Works combines machine translation and ChatGPT to improve accuracy. Bureau Works can identify parts of speech such as nouns and conduct reviews on parts of texts/whole sections as a whole.
ChatGPT integration by Smartcat
Smartcat is an all-in-one platform connecting businesses and translators into a streamlined content delivery loop. This presentation showcased the use of OpenAI for automatic translation, rephrasing, changing gender in translation, and supervised text processing.
The next phase is to provide more context in templates including comments, glossary terms, and fuzzy matches before releasing the products to our customer base. We’re also working on having a configurable UI, interactive suggestions, segment auto-labeling, and AI-based QA checks.
ChatGPT CAT by Terence Lewis
Terence Lewis built a dedicated ChatGPT CAT tool that is a gateway to several useful tasks: translation, paraphrasing, and summarization functions.
Key Trends
Industry researcher panel with Konstantin Dranch (Custom.MT), Florian Faes (Slator) and Jaap van der Meer (TAUS) reviewed the news and trends.
Corporate Approach towards LLM
Florian Faes
Managing Director at Slator
Key Takeaways:
- Launch of Hugging Chat – GPT alternative from Hugging Face.
- The News Wire – the first company to publicly declare using ChatGPT for translation at scale.
- The concept of document-level machine translations using LLMs means we must step up our game. There’s plenty of research on machine translations coming up from different places, including China. A case study in “mind your language” asked people whether they should integrate ChatGPT into their language service products.
- Reddit is charging for its API to LLMs because it holds the largest database of human conversations.
Current Limitations of AI
Jaap Van Der Meer
Founder at TAUS
Key Takeaways
- Hype it’s becoming hard to filter the real news from the fake. In addition, we’ve been talking to heads of big machine translation companies, and they’re wary of the LLM revolution, and it’s hard for them to tell whether six months from now they’ll still hold their jobs.
- Many companies have been affected financially by AI interruption. This is because a massive scale has changed the industry in the last 2 years, which is almost a paradigm shift, and we may need to watch and see where this heads in the coming years.
- More and more companies may have to switch to the MT-First translation strategy. This means that these LLMs won’t do everything independently and companies need to train them for better services (add their own knowledge and data). Ultimately, companies must have their data to handle and work with the LLMs.
- There’s a concern about whether LLMs may face issues such as quality prediction, AI quality control, and data privacy among others, and whether companies need to put this into consideration when switching to LLMs.
- Plenty of effort is going into training the models, and human involvement is still paramount. Even with the AI evolution, we can still hope that it will open up avenues for millions of jobs in translation and other industries such as customer service and marketing.
What localization people should do in the next quarter: The hype may take a while before it can settle. The best option is to go for the change and avoid staying behind.
Emerging Model Landscape
Advances in translation quality estimation with new LLMs are dramatic, concluded top minds in this field, Christian Federmann, Alon Lavie, and Stephen Lumenta, in a panel led by Olga Beregovaya. The forecast is that there will be significantly more use for fully automated translation without a human in the loop, with a large language model correcting errors made by machine translation.
Alon Lavie
VP of Language Technologies at Unbabel
Key Takeaways:
- LLMs didn’t just come out of nowhere, transformer models have been developed for neural MT. The biggest surprise has been how LLMs are suited to different scenarios and products.
- Regarding MTQE, Alon is excited about how LLMs allow us to easily conduct error detection and classification without the limited input that existed in the past.
- We are now in a window of time because some of these models can get wrong translations. But then, it is great that models like GPT-4 can autocorrect themselves when they run errors.
‘Prompt engineering may yield better results than other approaches.’
Christian Federman
Principal Research Manager at Microsoft
Key Takeaways:
- The shocking aspect of these LLMs is how well they do their job. The level of quality is high and this may mark a positive disruption.
- Prompt engineering may yield better results than other approaches.
- We will need more human input to verify GPT output, leading to an increased demand for human services.
Stephen Lumenta
CTO at Phrase
Key Takeaways:
- The biggest question with any technology regarding cost-benefit tradeoff is, “Just because ChatGPT-4 can do something, does it have to do it?”.
- New machine translation quality estimation is very impactful.
- There are still some regulatory constraints and stability issues with LLM developers like OpenAI and we may need to give them time to settle before we can risk any liability. The question around security is also high as we need to be conscious and responsible of what we expose our consumers to.
Action plan for localization managers
Industry researcher panel moderated by Konstantin Dranch and includes Anna Schlegel. Anna Schlegel co-founded Women in Localization and author of the popular guide “Truly Global.” In this session, she made a series of recommendations for corporate language managers to take advantage of LLMs.
Key Takeaways:
- Find allies in the upper management – the technology officer, the data officer and others are looking for implementation strategies, and localization directors can help with data and evaluations.
- Use LLMs for market research – find out regions where the company’s performance may be improved and brainstorm tactics such as local integrations, partnerships, and legislation to provide guidelines for the management.
- Hold GPT workshops to engage internal stakeholders.
- Test and pilot actively and share pilot results with peers.
Overall Takeaway: These measures will help localization managers improve their standing and position themselves strategically in today’s technology wave.
Conclusion
What Does the Future Hold?
Advances in translation quality estimation with new LLMs are dramatic, concluded top minds in this field, Christian Federmann, Alon Lavie, and Stephen Lumenta, in a panel led by Olga Beregovaya. The forecast is that there will be significantly more use for fully automated translation without a human in the loop, with an LLM correcting errors made by machine translation.
Comments are closed.