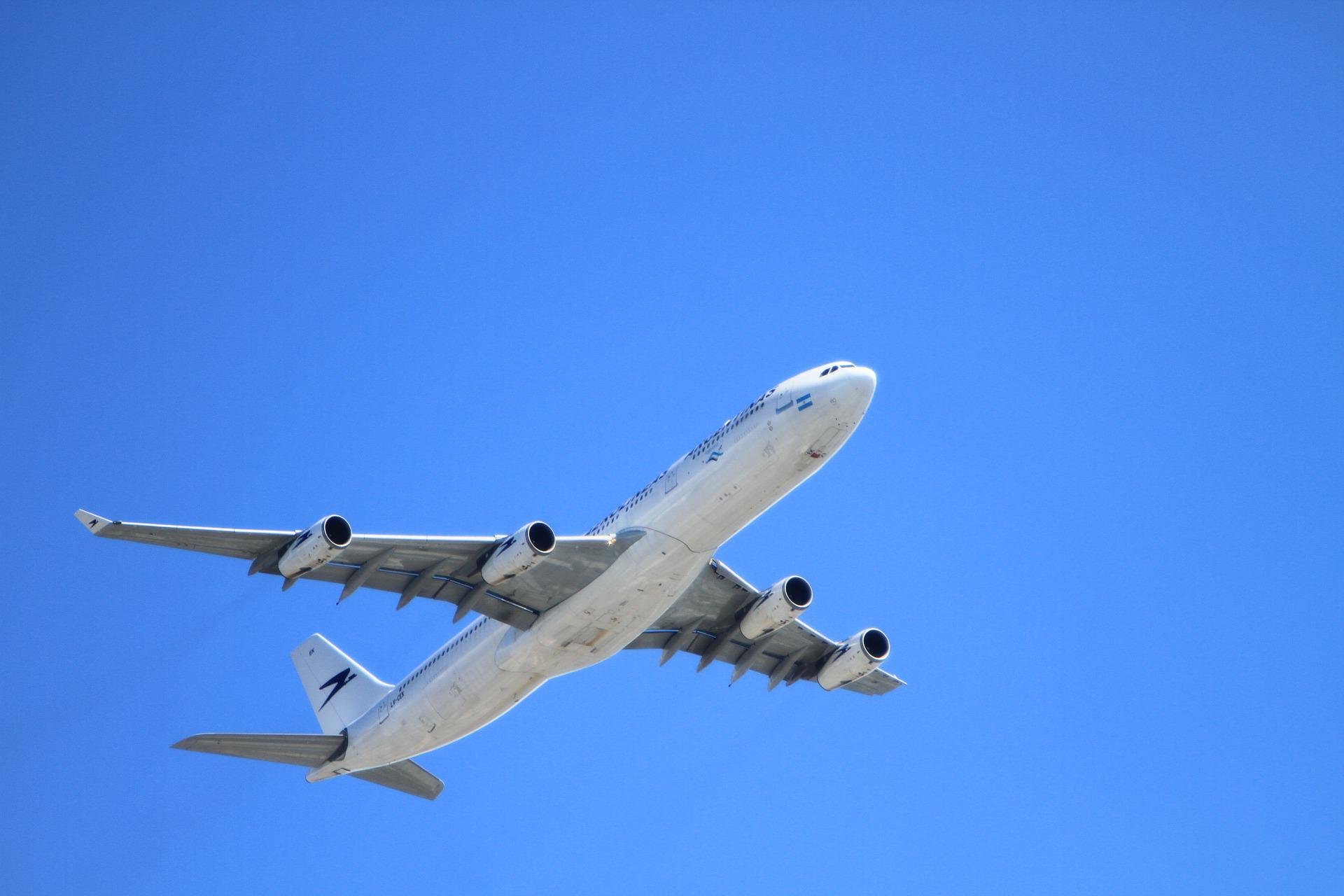
Engines from IT giants such as Google Translate, Microsoft, and Yandex often win in quality because search engine companies possess the whole internet as their data pool. However, with very specialized content and excellent translation memory, this advantage is nullified. In this case study, the engine from a smaller MT vendor Globalese won in all evaluations for Russian to English translation after gaining an enormous 115% boost to performance in training. We took advantage of the customer’s monster 1-million segment dataset accumulated over 10 years of consistent translations.
Language combination: Russian to English
Domain: Technical-Aviation
Training dataset: 1 million segments
Highest BLEU score attained: 51 (excellent)
Quality gains over stock Google: +67%
1. Dataset Preparation for Machine Translation
In this case study, we received a huge translation memory from the client, and processed it using our proprietary data pipeline. Our lexicographer kept aviation part names and nomenclature intact, and removed repetitions, segments flagged by automatic QA and inconsistencies. The resulting dataset for training has come out reduced by 60%.
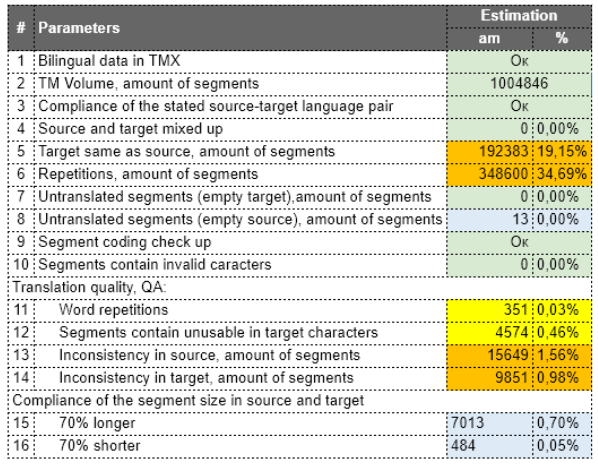
2. Training Machine Translation with TMX
Once we had a clean dataset, we train a set of engines with it, including Globalese, Google AutoML, Yandex, Amazon ACT, Microsoft Custom Translator, IBM Watson, and ModernMT Enterprise. The training took significant time and more investment than usual due to dataset size.
3. Machine Translation Automatic and Human Evaluation
It was worth the investment: training yielded huge improvements to BLEU scores and moderate improvements to hLEPOR scores.
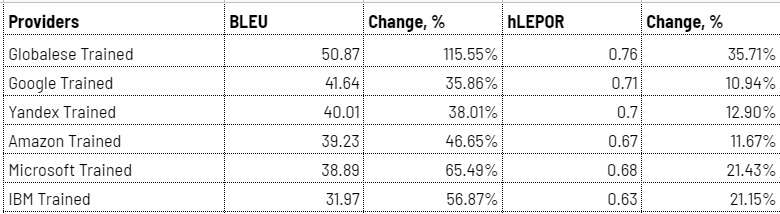
Globalese BLEU improved 115.5%, from 23.6 to almost 51, outstripping other engines in this experiment by 10 points or more.
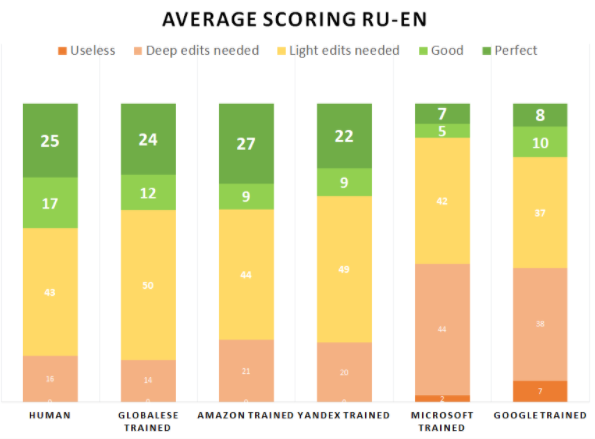
The human evaluation has been carried out as a blind test with three specialist linguists scoring and editing six engine outputs. As a result, Globalese won again, tied for the first place with Amazon ACT. In this exercise, scores correlated loosely with automated evaluations.
The client selected Globalese for further use due to the fact it was already integrated with their preferred translation software Memsource.
Overall human evaluation scores were moderate. The main reason was the fact Russian is an inflectional language, and many segments required suffix correction. Furthermore, the engine often misses some words in the sentence. So the linguists need to stay vigilant and apply a consistent cognitive effort when working on Russian to English MT.
We expect translators working with this engine to achieve editing speeds of 1000-1500 words or up to 4-6 pages per hour after a period of adaptation.
Case study by Konstantin Dranch.
Image credit: Ivan Lapyrin
Comments are closed.