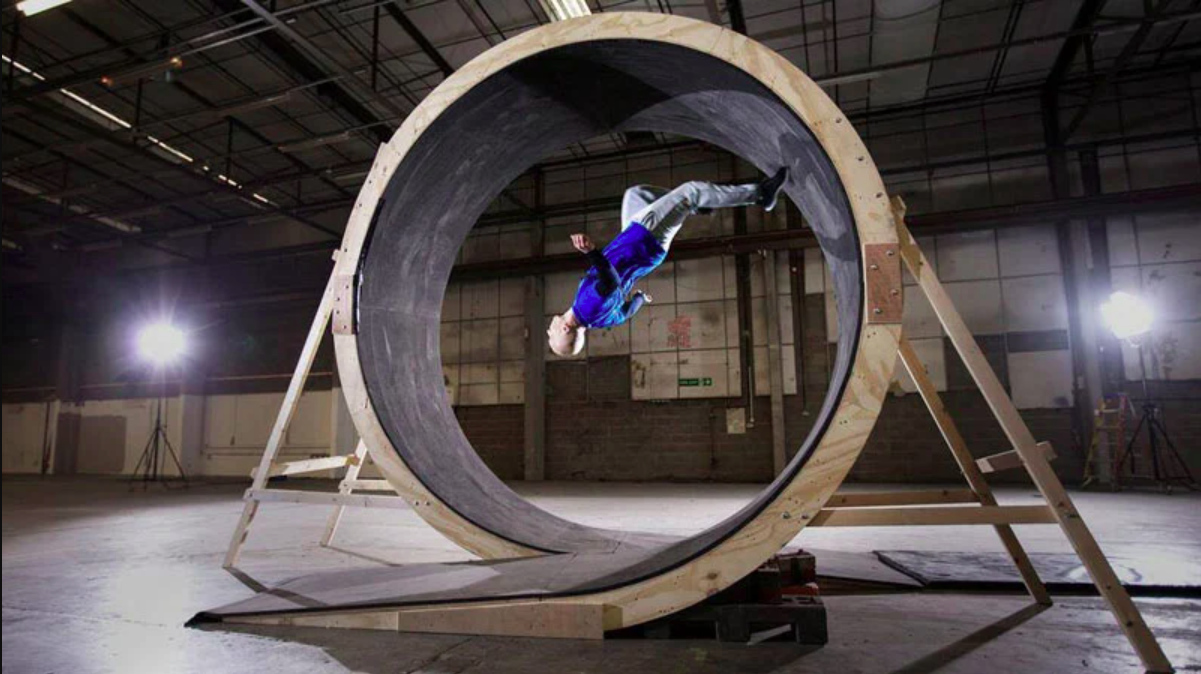
We’re seeing a rise in the number of projects and requests that rely on raw machine translation. When Custom.MT started, clients wanted to train models to improve the productivity of human translators, and to reduce the cost. Today, more than half of the requests we get are about doing the translation fully automatically, without a human in the loop reviewing the output.
In the past, fully automatic translation had been the domain of large very technical organizations with a vast ocean of content. Think Dell, eBay, or Booking.com. Today’s news is the level of involvement from medium-sized organizations. There is a definite increase in demand: eLearning courses, websites, videos, shop inventory descriptions, support chat.
Language managers are getting more audacious with the machine. Here are a couple of fresh cases to illustrate.
European Food Safety Authority
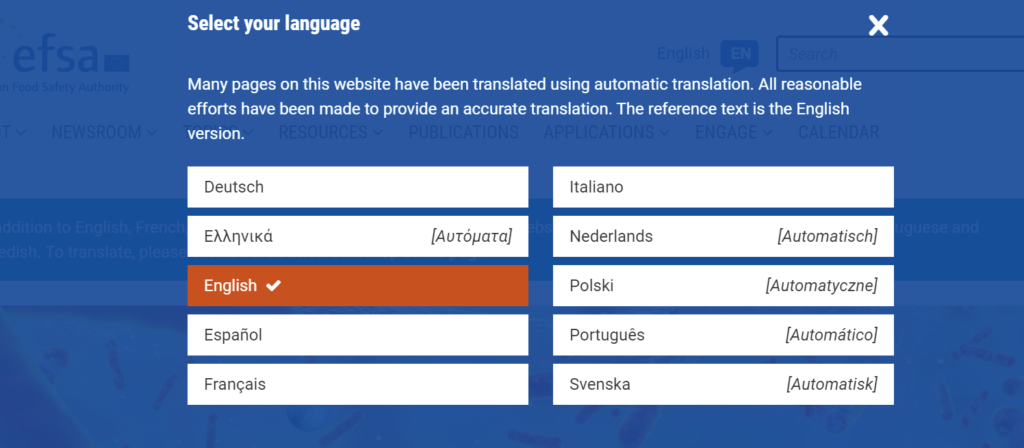
A year in the making, the project to make the EFSA website more multilingual and present news in more European languages culminated with the integration of eTranslation, the European Commission’s MT service.
Despite having a well-staffed internal linguistic team, and keeping in mind that announcements about food safety regulations are a type of content that one might call sensitive, the agency opted for the automated process.
The reasoning is clear: to eventually cover more of the 24 EU languages. Plus, eTranslation is free for EU institutions and the internal translation team has the ability to monitor quality and help retrain models.
World Health Organization
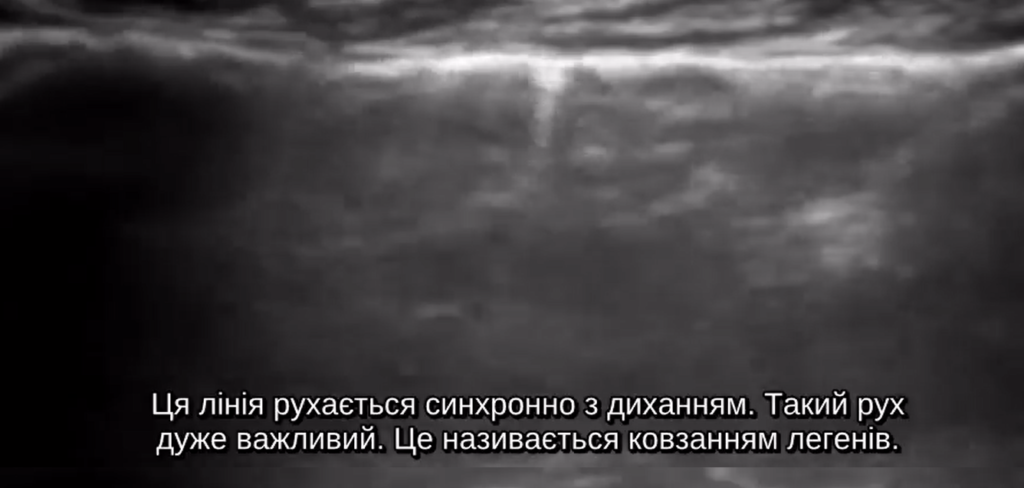
To narrate eLearning videos in Ukrainian, the World Health Organization computational linguist Mirko Plitt created a workflow where the voice is generated automatically via Azure text-to-speech. Mirko added a hack to make the AI voice sound more natural by inserting silences in-between the utterances based on timestamps and audio file durations.
“We need to do better than subtitles only. Now, what’s the easiest step up? Speech generation!” – explained Mirko.
Although it is still possible to discern that it is the bot speaking, and not a real person, on the whole, the narration is clear, coherent, and – yes – automated.
Red Hat eLearning Courses
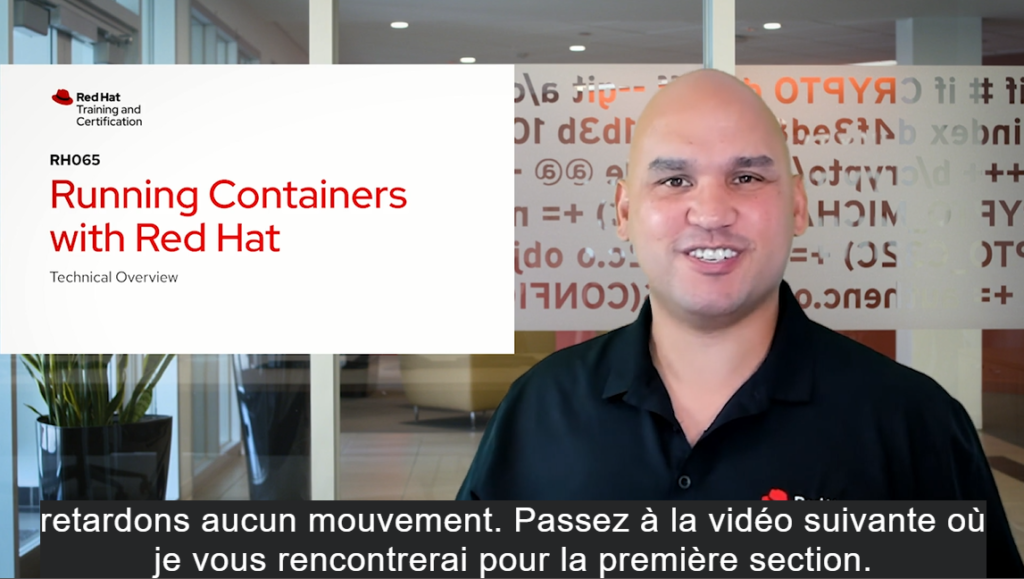
The IT giant’s Academy presents its material to train engineers in nine languages, and liberally uses video. The course videos are narrated in English with great energy and enthusiasm by Red Hat’s presenters, and the subtitles use machine translation for language coverage.
While the course books go through a rigorous review by experts in software, a lot of the subtitles accompanying videos are generated automatically, so Red Hat students have the benefit of following the video material in their language, and the video production process is very fast and cost-efficient.
When Machine-Only is Feasible
Going full-on machine makes sense when speed, affordability, and scale are more important than linguistic quality. This covers the following scenarios:
User-generated content
- Chat (Drift, Intercom)
- Social media posts
- Reviews
- Forums
- Marketplace listings
Massive knowledgebases and eLearning platforms
- Help files
- Manuals and guides
- Wiki pages
- Corporate learning courses
Secondary marketing materials
- Blog posts and announcements – to make them available in additional languages
- Website pages with low view count – to get them indexed by search in additional languages
- Product descriptions
Machine translation is not suitable for conveying the same emotion as the original text. It also should not be used for high-stakes documentation such as clinical trial results. For everything where understanding the gist is sufficient, it will fly. It has been proved by CSA Research that for the user experience and for traffic generation, MT even with errors is much better than the absence of translation.
Just remember to include a disclaimer “machine translation” to set user expectations.
Only Useful When Integrated
In a day-to-day scenario, one can copy and paste the text into free Google Translate or DeepL piece by piece. With multiple languages, groups of people, complex formats, and hundreds of thousands of items to translate there must be integration and a continuous flow of content. Manual is no longer enough.
Integrating via a TMS. Translation management systems (TMS) come with connectors to content management systems on one side and connectors to MT on the other. They are a natural bridge between localization and content. Localization technicians can set up the link simply by configuring a template, without any coding.
However, routing MT through a TMS can also decrease translation quality. TMS uses translation memory technology to break up texts into sentences (segments) to help linguists by recalling past translations from a database. When sent to MT, individual sentences carry little context. The AI model sees only a small part of the text, and it becomes hard for it to classify the domain, and maintain terminology consistency.
Direct integration. A better approach is to send the translations to MT in bulk, with the whole page or document. This way, the AI model has more data to identify the right translation.
So, if integrating MT via a TMS, first check with the vendor whether the text can be de-segmented and re-segmented for the benefit of the MT model and the human linguist. If that functionality is not supported, consider ditching the TMS and integrating MT directly.
Improving Accuracy
If working in a specialist domain with lots of terms and a professional or scientific lingo, you can significantly improve model accuracy with training.
Model training requires data: preferably, several million words of high-quality translations relevant to the topic. We see minor improvements when customizing a model with datasets of just 0.2 million words, and tremendous gains from adding 3-4 million words.
The training data can come from various sources:
- Organization’s translation team and suppliers
- Parsed online from similar websites
- Acquired on the data marketplace (3,000 – 4,000 euros per million words)
A glossary is another way to tailor MT output. Glossaries can be constituted manually ahead of the project, or semi-automatically by combining the extraction of high-frequency words from the company’s website with expert linguist work.
In some fields such as medical, finance, crypto, and legal proceedings, there are existing assembled glossaries of industry terms. An engineer can use those to make the engine compliant with the standard in the field.
Crowdsourced Review
The past five years gave rise to crowdsourcing platforms like MTurk and Toloka. With thousands of people registered there for linguistic work, it’s possible to access a paid crowd of bilinguals via an API at any time of the day.
Professional translators shine in projects that require specialist knowledge in the technical, medical, legal, marketing, or scientific domains. Bilinguals are a fit for less complete and consumer-facing content. There they can effectively spot the obvious and embarrassing mistakes of machine translation.
The advantages are availability and cost. Compared to the professional translator review fee of $0.04 – 0.08 per word, validation by bilinguals can cost as low as $0.01-0.02.
Linguist Role
Pure MT workflow phases out linguists from reviewing each individual item of content to win in speed, instantaneous availability, and cost.
However, that doesn’t mean that the need for a linguist disappears. She or he can be very involved in gradually increasing the accuracy of the AI model. For example, by spot-checking content after publication.
The important parts to monitor are:
- Highly-visible content: page headers, top-read pages, names, and definitions
- Tone of voice
- Language inclusivity
- Introducing new definitions and expanding the brand language.
The concept of reviewing MT after publication and only where it is necessary has been introduced by Microsoft’s Chris Wendt almost 10 years under “Post-Publish-Post-Editing” or P3 and has been effectively productized by innovative companies such as Unbabel.
With this approach, the linguist takes on the role of the MT engineer and cultural advisor.
Conclusion
Pure integrated MT is the only way to feasibly translate user-created content or reach a scale beyond 50 or 100 languages. New languages and unofficial content are the best places to start introducing pure MT. With annual increases in MT accuracy, and with the ability to customize and fine-tune models, more important content can be automatically translated.
Photo credit: twistedsifter.com, Damian Walters completes world’s first loop-the-loop on foot
Comments are closed.