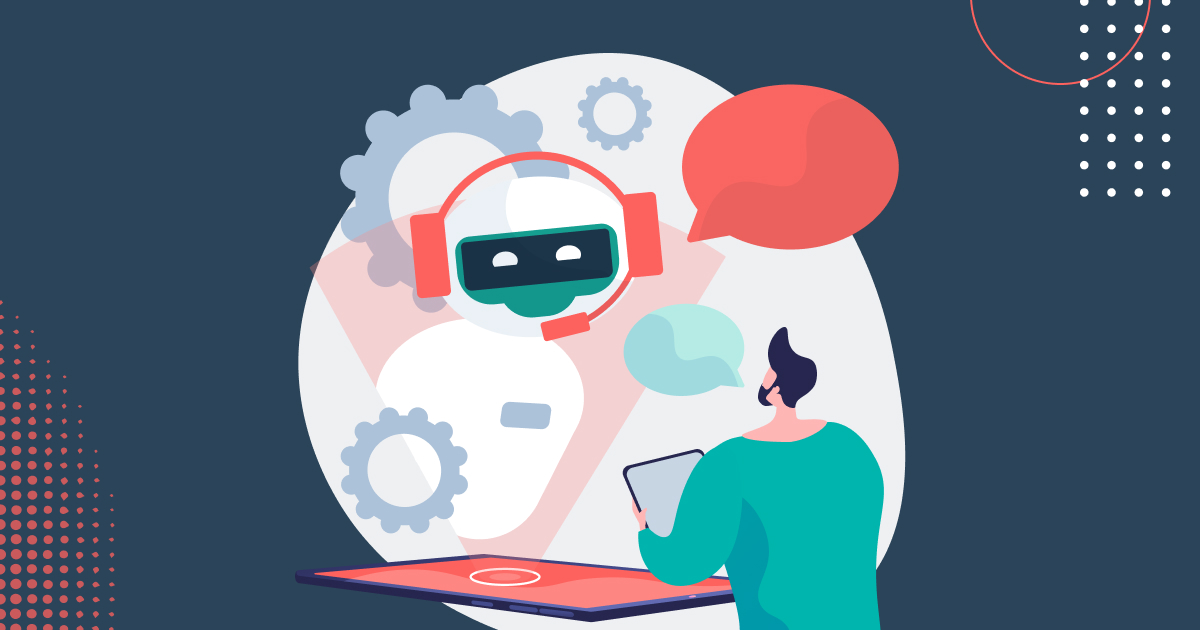
With the impact ChatGPT had on localization industry and most other fields, millions of people learned that computers can already communicate and brainstorm better than most humans. Businesses are now aware of its capabilities, while AI labs roll out a much bigger GPT-4 model. I offer you an early outline the impact of this technology on localization.
As of 2023, the language services market is a multi-layered cake with different mixes of expert human work and technology on each level.
At the bottom level, machine translation makes 99,99% of the world’s translations, more than 2 trillion words per day in apps, information monitoring systems, and browsers. However, the machines get only 2% of the money.
In the middle layer, professional human translation takes more than 90% of the spend, while processing less than 0.01% of volume in words. Furthermore, over 2/3rds of human translation is already a revision of machine output, and the share of machine-aided work is growing.
At the top, there is transcreation, a creative rendition of slogans, songs, subtitles, and other artistic content where the emotional impact is important. Transcreation is about reinventing the text in another language, possibly using completely new phrases. This kind of work has been very pricy in comparison to translation in the past.
Let’s look at the impact on each level.
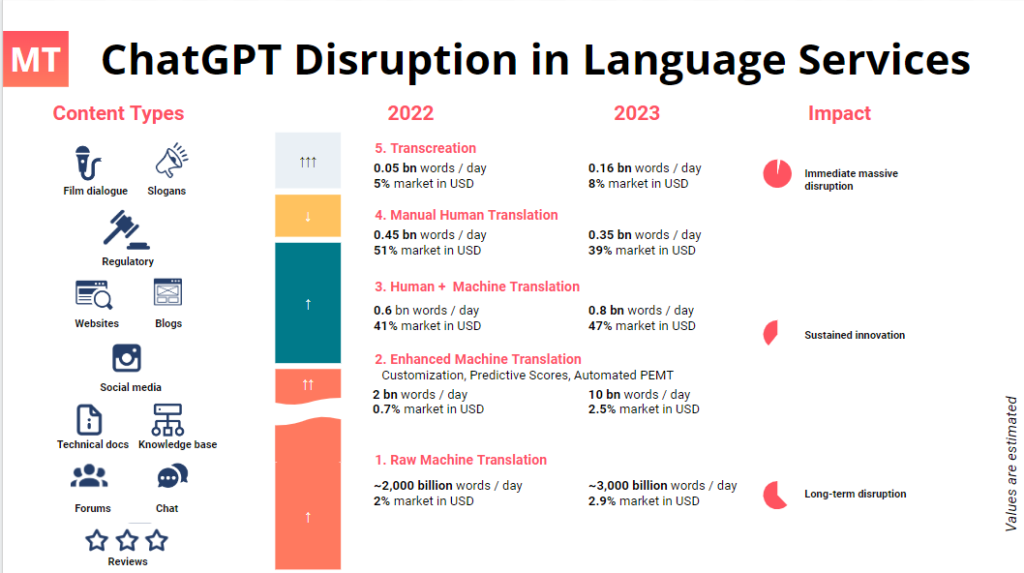
ChatGPT Revolutionizes Transcreation
This is where ChatGPT hits the strongest. AI is now able to write passable slogans, poems, and songs, and create word plays from a given prompt. Writers and even school children are already using ChatGPT to generate drafts that they can edit in a process similar to how machine translation post-editing. Speaking of the impact on localization industry, the onus is now on businesses to adopt ChatGPT at scale to increase the speed and reduce the cost of content production, running a human review and fact-checking.
In the past, transcreation was done manually using tools like Microsoft Word and Google Docs. And now generative models can be a part of these tools, functioning as autosuggest.
Transcreators will be able to produce 2-4 times more content per hour by giving prompts and reviewing the output. The productivity of transcreation will translate into a lower cost, and I forecast at least 2x growth of the service volume, perhaps 3-4x growth in the next two years.
There will be resistance and some institutions will ban AI copywriting, for example, the ICML conference has already forbidden papers written by ChatGPT. In the societal split into technocrats and Luddites, transcreation and copywriting will become divided into “artisanal” and “machine-aided” categories, similar to translation and post-editing, with the latter seeing a significant increase in volume but potentially slower financial growth due to corporate budget resilience. Even if copywriting becomes more affordable, it doesn’t mean marketing departments will suddenly get more money for it.
Takeaway: copywriting and transcreation will be transformed by GPT, with an explosion of volume in words, and a slower growth in budgets.
Impact on Professional Translation
In comparison, the influence on professional translation will be more subtle. Rather than disrupting, ChatGPT and other GPTs are likely to impact the industry in a way that drives sustained innovation and gradually reduces the amount of human labor required for revision of translation and localization. Even with the assistance of ChatGPT and other language generation models, it will still be necessary to fact-check and review translations in high-risk scenarios, and there may be resistance from both humans and businesses to fully rely on these models.
Once an API for GPT-4 becomes available, their capabilities will be built into CAT tools and TMS in a number of different ways: translation, text quality optimization, scoring, and fuzzy match repair. This will lead to a decrease in the share of words that need to be reviewed by translators and an increase in the number of words a person can review per hour. Imagine Smartling, Phrase, XTM, memoQ, and RWS Trados, all integrating GPT in some way.
It is possible that in 2 years, the average translator will be able to achieve a productivity of 1,500-2,000 words per hour when supported by the latest AI up from the current range of 450-900 words per hour.
Conclusion: seeing a gradual bump in productivity, the market for professional translation remains in the same hands that control it today.
In Value-Added Machine Translation Programs
By a Machine Translation Program I mean a business operation where an expert linguistic team oversees machine translation and boosts its quality using add-ons: automated post-editing, domain adaptation & customization, and predictive scoring.
The level of accuracy achieved is sufficient for specialist support chat, knowledge bases, technical docs, internal communications, product descriptions in online stores, eLearning courses, and documentation packs.
Large language models have multiple applications in this area, starting with the fidelity of translation in inflective languages, fixing fuzzy translations, and rewriting poor-quality source text to make it easier to translate.
Impact on Raw Machine Translation
It might come as a surprise, ChatGPT can translate with great quality in many languages! The question is whether we will be using instead of mainstay translation tools. The first experiments by non-professional evaluators instantly proclaimed ChatGPT dominance vs Google Translate and DeepL, and in the translation industry, linguists started using ChatGPT for Chinese also with positive feedback.
Large language models have distinct advantages over conventional neural MT systems. Their flexibility allows the user to change tone and voice and make personalized translations for different audiences. For example, it’s possible to prompt it to “translate for a 5-year old”, or “eliminate gender bias from the following translation <…>”. They also learn from source content faster, eliminating the need to maintain a fleet of MT models.
The main disadvantage of ChatGPT for machine translation is its infrastructure cost. Where an MT model can run on a single CPU or a GPU, running a large language model requires a multi-GPU setup, at an eye-watering cost, and only big tech will be able to afford to do so at scale. Further, organizationally the switch will not be easy to pull off in larger organizations. Enterprises might take years to switch to ChatGPT for machine translation due to security concerns and their past investments in neural machine translation systems. Even though you can translate with ChatGPT, you won’t be allowed to.
Conclusion: Large language models like ChatGPT represent a new generation of technology in machine translation, but because translation is an established and well-serviced market, the change here will be not as fast or pronounced as in copywriting.
What to do in 2023 if you’re a localization director? Start GPT pilots in content production, and transcreation where the impact is immediate. A lot will change before the year is over.
Comments are closed.