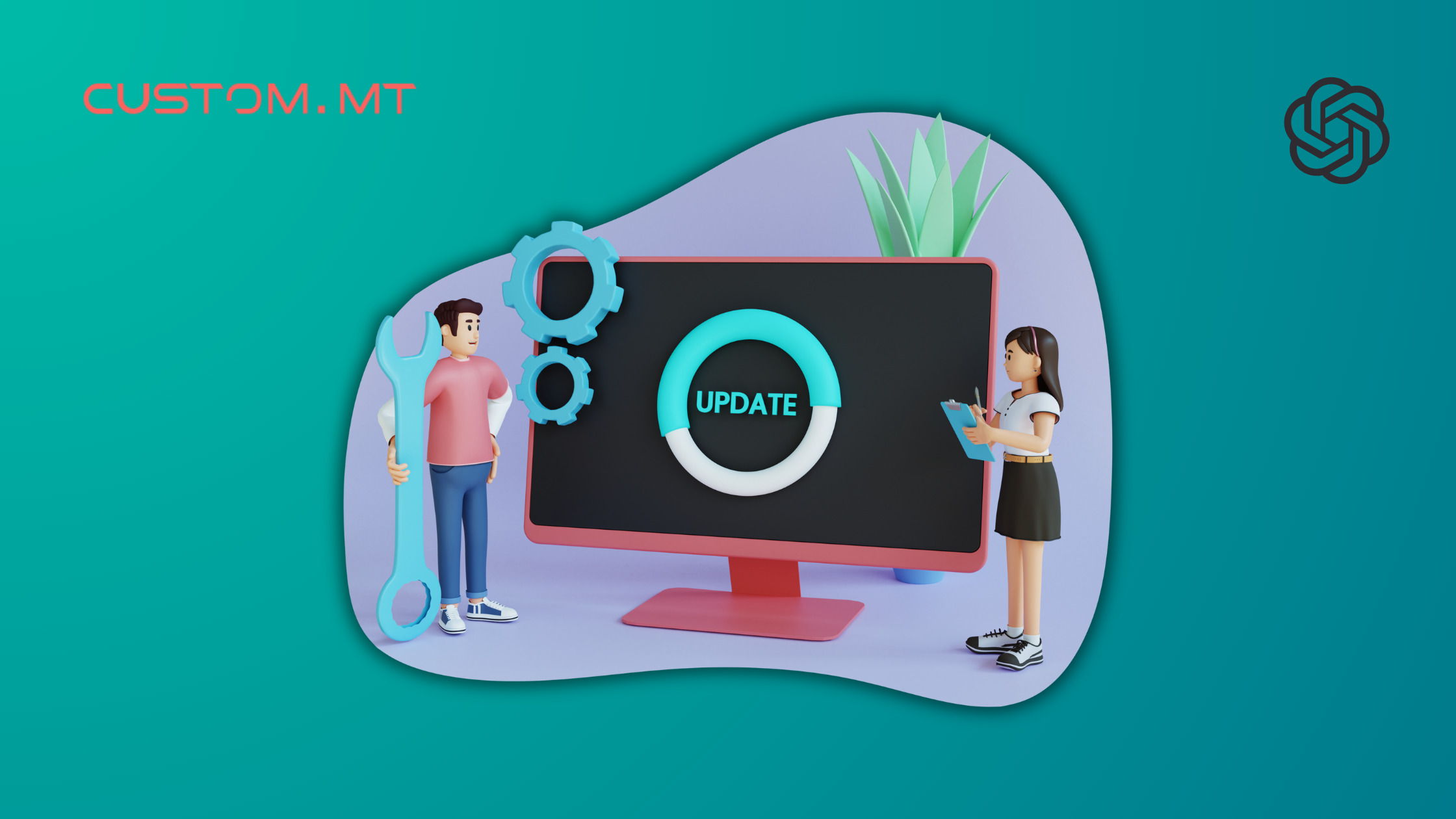
On Monday, November the 6th, OpenAI unveiled improved models:
- GPT-4 Turbo with a 3x lower price and 16x larger context window
- GPT-4 Vision availability in API
- Synthetic voice, speech, and vision in prompts
- Ability to create agents
- Tools to get repeatable generation
- And more
The release is a gauntlet of challenge thrown to a broad range of AI companies, not just LLM builders like Antropic and Cohere, but also speech recognition, synthetic voice, and machine translation companies. OpenAI positioned itself as a future one-stop shop for models.
While getting image, voice, speech, and text AI processing from a single source and having all data handled by one organization is a worrying prospect, localization stakeholders stand to benefit from the intensifying competition in GenAI. Here is how:
Translating Image PDFs
With vision and text capabilities from a single source, it is possible to tackle a perennial problem in translation – image PDFs, including document scans, phone photos of invoices and receipts. Moreover, the model can extract information from the receipts and understand it.
For the test, our developer Martin developed a script that was able to take a receipt from lunch in a Czech restaurant, extract all information from it, and translate it into English. It took less than 20 minutes.
Yum!
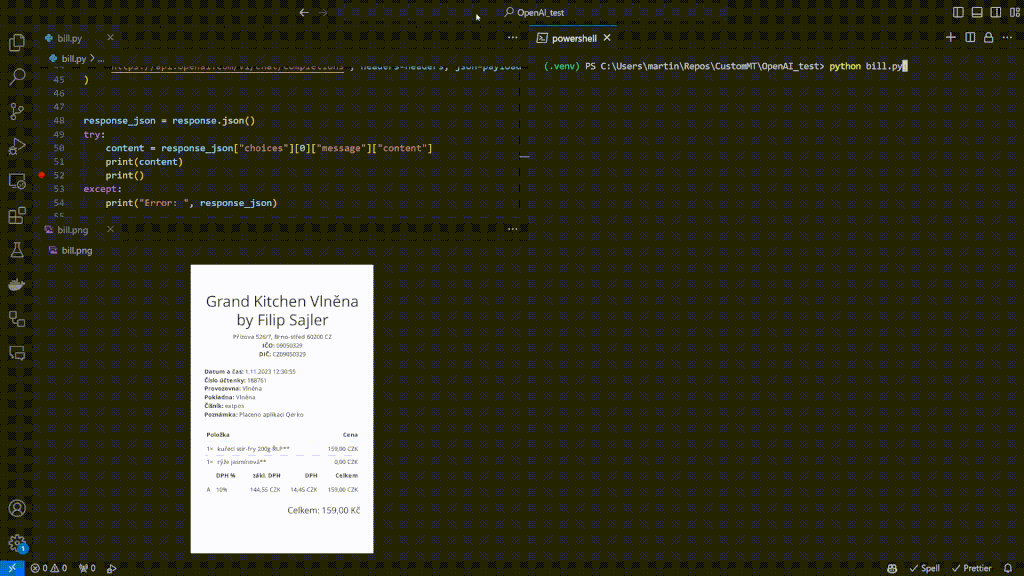
Automated Localization Testing for Screenshots
Localization testing application of user interfaces in multiple languages is the next problem localization PMs may attempt to automate with GPT-4 Vision. Today, program managers responsible for UI translation take screenshots manually or automatically and then pass them to linguists to verify if the text fits the boxes, and if it reads right in the target language.
UI localization testing is labor-intensive, but it is paramount to getting a smooth user experience across multiple locales. With a vision/text model operated via a prompt, localization managers will be able to automate the testing and highlight potential issues.
We’ve run an experiment with the GPT-4 vision in preview by fabricating bugs in our own login screen (screenshot below). The model identified them correctly:
1. Text Overflow: The button labeled “Přihlásit se do tohoto krásného nástroje” has a text overflow issue where the text is too long for the button size and breaks out of the button boundaries. This could lead to problems with readability and may affect user interaction.
2. Text Consistency: The language used in the button mentioned above is Czech (“Přihlásit se” means “Log in” in Czech), which is inconsistent with the rest of the interface that appears to be in English (e.g., “Email”, “Password”, “Not registered? Create an account now”). This inconsistency could confuse users.
3. Incomplete Localization: If this screen is intended for Czech users, all visible text should be localized to Czech. Key phrases such as “Authorization,” “Email,” “Password,” “I forgot my password,” and “Not registered? Create an account now” should be translated to maintain consistency and ensure user comprehension.
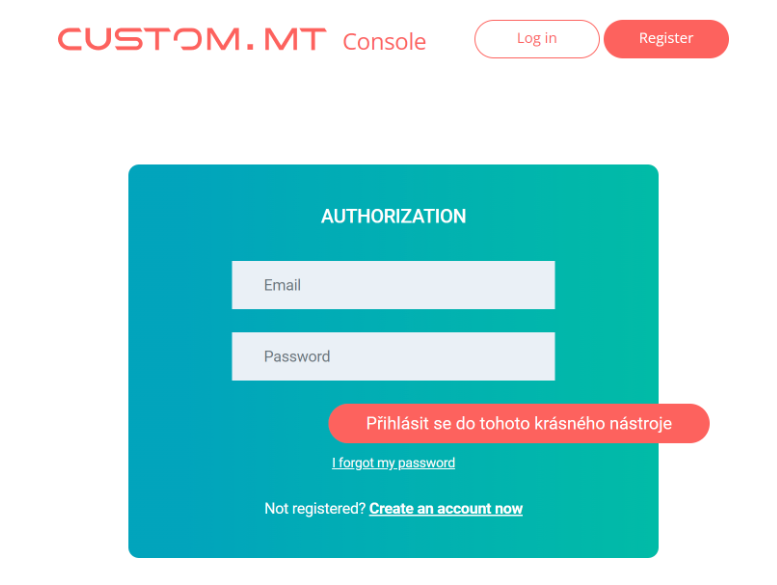
GPT-4 Turbo at the price of NMT
GPT-4 Turbo prices are 3x lower than GPT-4.
In the past months, many localization stakeholders in our network experimented with GPT-3.5 Tubro and GPT-4 models. While they found GPT-4 superior in quality, they couldn’t often use it with glossaries and detailed style guide instructions due to a high cost. Long prompts applied on a per-segment basis in CAT tools and TMS caused expenses to soar up to $0.15 per segment, which is close to manual translation costs. Other issues with the model were speed and reliability. Managers of large-scale localization programs reverted back to classic machine translation after experimenting with OpenAI. Only the most persistent and optimistic ones carried on despite setbacks.
With a move to reduce price, it feels like the setbacks are temporary, and that GPT may replace NMT in the observable future. The price of GPT-4 Turbo applied with a glossary and a style guide in the prompt should be on par with stock machine translation models like DeepL, Google Translate, and Microsoft Translator.
It is becoming easier and in some cases more viable to customize translation for style guide compliance with a prompt, than to fine-tune models such as Google Translate AutoML, or Microsoft Custom Translator.
Service | USD per million characters |
GPT-4 Turbo Document translation, no CAT/TMS | $3 |
DeepL | $27 |
GPT-4 TurboSegment translation in a CAT/TMS | $30 |
Google Translate AutoMLFine-tuned | $80 |
GPT-4Document translation, CAT/TMS short prompts | $60-120 |
GPT-4 maxed-out glossary applied to tiny segments | $22,350 |
NB! Speed with GPT-4 remains an issue, especially at scale. Until OpenAI addresses speed, we expect the utility of the model in large-scale scenarios to remain limited.
Automated Audio Description
Less than 24 hours after GPT-4 Vision & Voice, a developer built an automated sports commentator by passing every frame of a football video via a simple prompt to generate a narration.
A demo of AI video narration has already been showcased on the OpenAI cookbook. As a proof of concept, it clearly shows that videos can have audio descriptions fully automatically at a low cost.
The ease of building video narration and audio description may be deceptive – it will actually take months to get the technology working reliably. We believe that audio descriptions for prime-time shows and theatrical releases of Hollywood content will remain manual, going at the average rate of $27 per minute. The rest of the market might go for an embedded AD at a fraction of the cost.
Afterword – Will Anything in AI Stay Defensible?
OpenAI cast a wide net across different niches and boxes of the growing AI industry. Previously seen as a chatbot builder, it now will be perceived as a threat to thousands of startups, including the full extent of language technology providers.
- Automated video dubbing companies
- Machine translation providers
- Speech recognition providers
- Text & video analytics
- AI quality assurance
In the past, any language tech startup would hear “How are you better than Google Translate?” In 2024, the question is rapidly turning into “Wouldn’t OpenAI do all that in 1-2 minutes?” Entrepreneurs and buy-side localization program managers, prepare to show how specialized systems built for localization are the only way to completely address business needs. It’s a far cry from a minute-made prototype to a working solution.
PS: at the time of writing this, OpenAI’s API went down in a major outage. The doom of AI companies was averted – for today, at least 🙂
Comments are closed.